[S1.06] AI Platform as a Service
![[S1.06] AI Platform as a Service](/blog/content/images/size/w1200/2024/07/S1.-05.png)
"The platform of services is as big as the world. It is never overcrowded."
- Mahatma Gandhi, an Indian lawyer, politician, social activist, and writer who became the leader of the nationalist movement against the British rule of India. He was also a prolific writer and thinker, writing extensively on topics like nonviolence, civil disobedience, and social justice.
Platform as a service (PaaS) or application platform as a service (aPaaS) or platform-based service is a category of Cloud computing services that allows customers to provision, instantiate, run, and manage a modular bundle comprising a computing platform and one or more applications, without the complexity of building and maintaining the infrastructure typically associated with developing and launching the application(s), and to allow developers to create, develop, and package such software bundles.
The first public platform as a service was Zimki, launched by Fotango, a London-based company owned by Canon Europe. It was developed in 2005, had a beta launch in March 2006 and a public launch at EuroOSCON in 2006. At the time of its closure, Zimki had several thousand developer accounts. It had demonstrated the technical viability of Platform as a Service, but also provided the first example of the perils of being dependent on a single provide.
This topic is the 6th topic of our series of AI Business Models, with a detailed guide and explanation.
There may be difficult to some people to capture the term of Platform as a Service, if yes, please revisit our blog S1.01: AI Business Models Overview to capture more detail about this term.
AI Business Model Blog Series
After years serving our client in the AI domain, we are impressed about many different AI approaches on products / services from our client. So we love to classify them as a Blog Series for AI Business Model, our 1st Blog Series (S1) to bring a summary on this topic and share to any of AI founder, entrepreneur out-there who dream to build their own AI kingdom. Some may already having their business but they can also refer on the market research to grow their AI business.
Series name: AI Business Models, a detail guide and explanation
Series blogs:
- S1.01: AI Business Models Overview
- S1.02: AI Education Business Model
- S1.03: AI Consultant Business Model
- S1.04: AI Development Agency Business Model
- S1.05: AI Software as a Service (AI SaaS)
- S1.06: AI Platform as a Service (AI PaaS) (this blog)
- S1.07: AI Infrastructure as a Service (AI IaaS)
- S1.08: AI Business Models transition possibilities
Story of "Soonicorn" AssemblyAI
Like other business startups, AI PaaS comes from Idea to Minicorn (valuation from $1M), to Soonicorn (soon-to-be unicorn, in the middle), and then reaches to be Unicorn (valuation from $1B). Focusing on speech-to-text solutions, AssemblyAI is one of the very successful examples of AI PaaS.
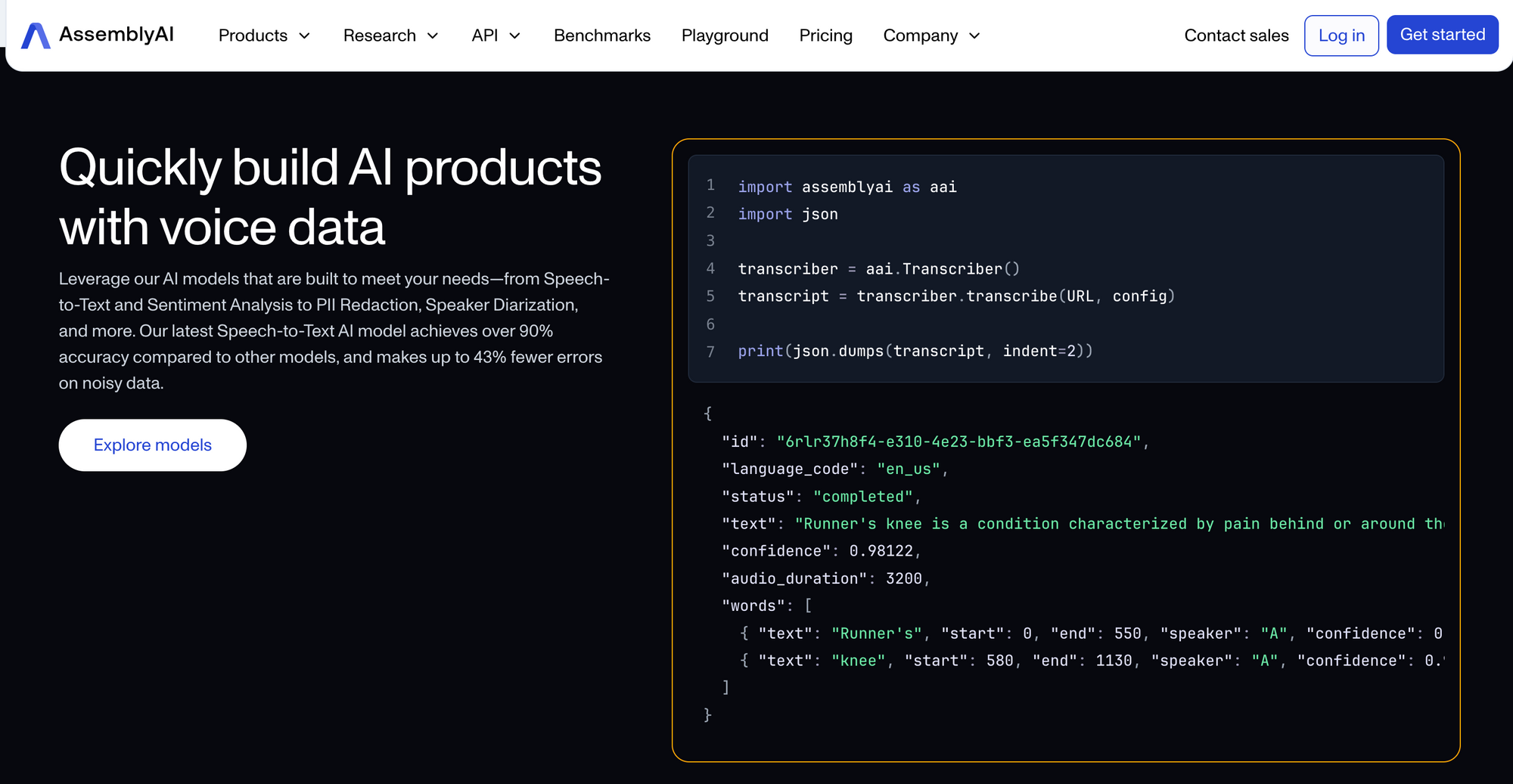
Similar to AI SaaS, AI PaaS also be built with an AI Model as a core feature. In the case of AssemblyAI, their core feature is the AI Model which helps to transform speech (voice) to text, so the focus is more in general technical solutions. This solution will then be used by another companies to apply in their specific solutions, products where speech to text is integrated to solve one specific feature.
The success raised $115M in 04 funding rounds, being used by "BBC News" are 02 of the remarkable achievements of AssemblyAI.
Basic about PaaS
AI PaaS solutions are designed to help developers build products that use machine learning (ML) and deep learning (DL) faster and with less effort.
PaaS Environment
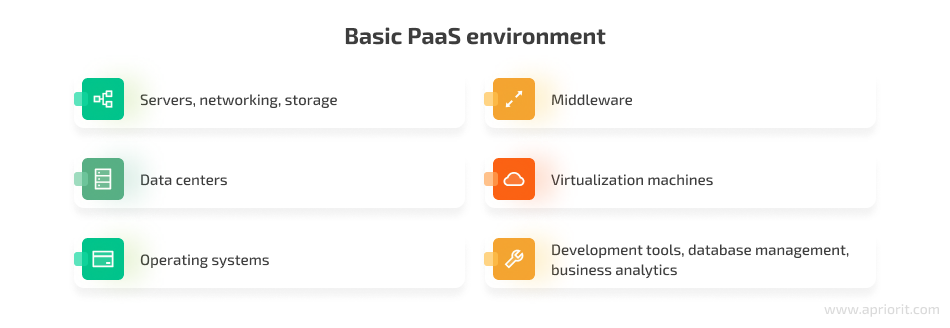
With PaaS, a cloud vendor provides an environment for building, deploying, and maintaining applications. A basic PaaS environment usually includes two main groups of components required for application development: infrastructure and a platform.
AI itself is all about processing enormous amounts of data, which, in turn, requires extensive computing power. This is why, similar to the standard PaaS model, many AI service providers offer infrastructure resources, computing resources, and virtualization capabilities. All the big data required for training ML models and improving AI solutions needs to be stored somewhere.
Components of AI PaaS
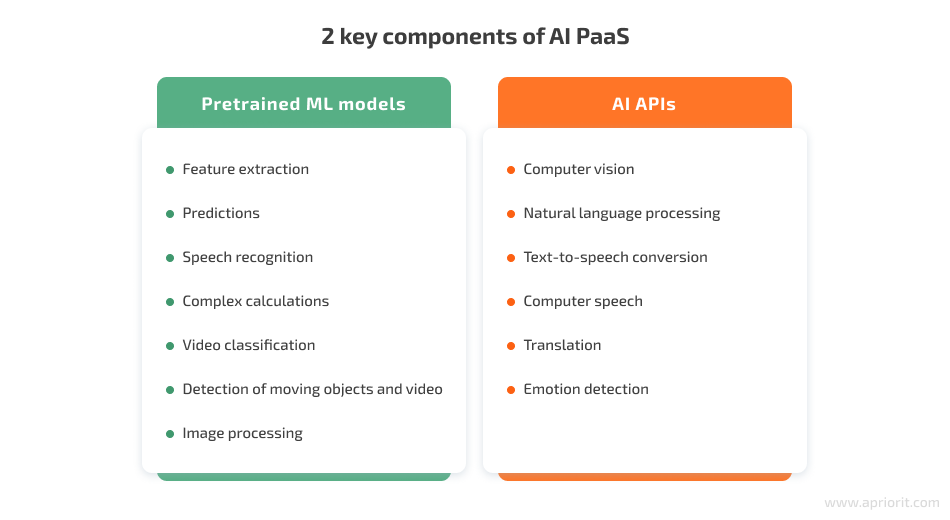
ML algorithms and models play the main role in implementing AI-based solutions: they process and analyze data, solve specific tasks, and provide the end result you expect. However, building and training machine learning and deep learning models from scratch requires enormous resources and expertise. This is why many cloud vendors offer pre-trained models and algorithms.
Application programming interfaces (APIs) make it even easier to implement AI functionalities in your application. Some vendors provide ready-to-use APIs for various AI functionalities as part of their platforms or as an independent service. AssemblyAI is an example of AI APIs.
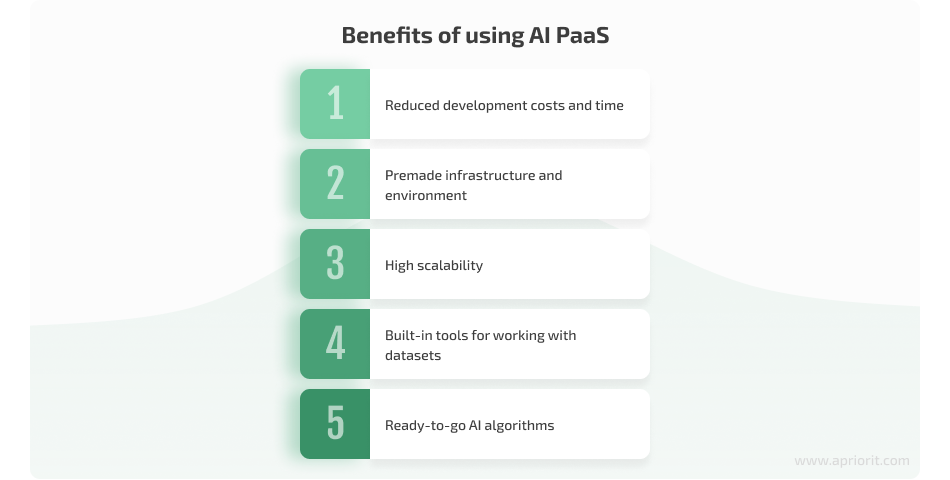
Highlight on market
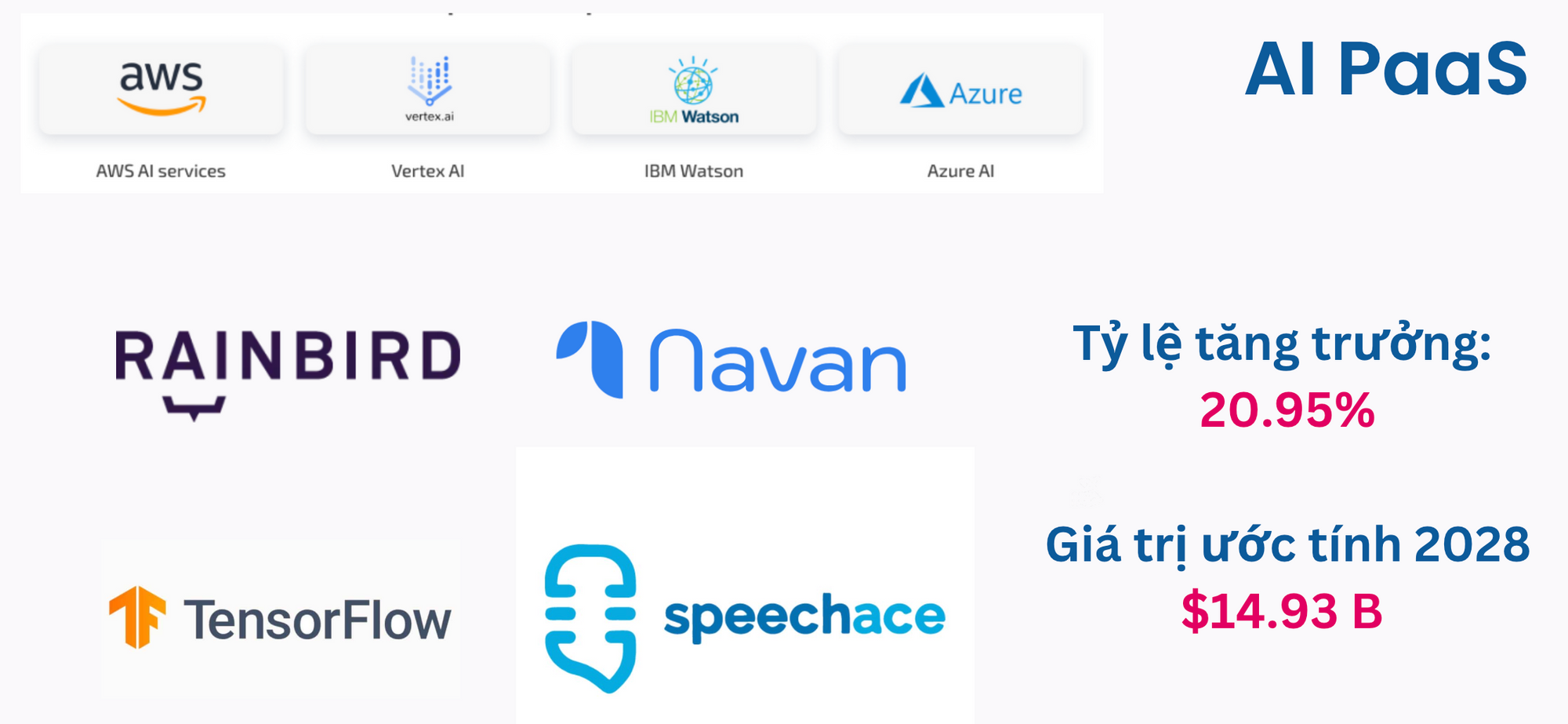
Referring to the high-level statistics of this business model, we can see a big CAGR (considering a market growth rate) of 21%, about half of AI SaaS CAGR, with the value estimated $15B in 2028, target of about $22B in 2030, equal to 40% AI Education and 1.6% compared to AI SaaS.
This is a very competitive market because of the presence of 04 tech giants: AWS, Google (Vertex AI), IBM and Microsoft (Azure). Based on the great advantages of cloud services, the 04 tech giants continue their big investment on AI PaaS solutions, both with Pre-trained models and AI APIs.
Action Recommendation
AI is again a very large topic, so AI PaaS working in this field always is very challenging, especially in competing with the 04 big tech giants. On the other hand, once your business is settled and welcomed by your clients, the business is more sustainable.
- Condition to start: the most important of AI PaaS is the specialty of your AI Model solution. So deep in technical, you must select a very specific technical problem and build your solution which must be the top solution in the field.
- Condition to scale: finding the best use cases, and markets that utilize your solution the most, then from there searching for all clients in those fields. Moving from use-case to use-case is the best approach.
- Condition to success: build your brand as the top solution for your technical problem, tailoring your solution to satisfy a lot of different use cases, and businesses in the market.
- Skill needs: deep know-how in the technical problem.
Ready to start your own AI PaaS Business? From Investidea, we would love to talk more with other AI PaaS firms to open an AI Alliance. Together, we can fulfill the diversification of technologies, domains, and user cases, which can help us to grow together, and serve our clients better.
Comments ()